Deep Learning In Computational Mechanics
Download Deep Learning In Computational Mechanics full books in PDF, epub, and Kindle. Read online free Deep Learning In Computational Mechanics ebook anywhere anytime directly on your device. Fast Download speed and no annoying ads. We cannot guarantee that every ebooks is available!
Deep Learning in Computational Mechanics
Author | : Stefan Kollmannsberger,Davide D'Angella,Moritz Jokeit,Leon Herrmann |
Publsiher | : Springer Nature |
Total Pages | : 108 |
Release | : 2021-08-05 |
Genre | : Technology & Engineering |
ISBN | : 9783030765873 |
Download Deep Learning in Computational Mechanics Book in PDF, Epub and Kindle
This book provides a first course on deep learning in computational mechanics. The book starts with a short introduction to machine learning’s fundamental concepts before neural networks are explained thoroughly. It then provides an overview of current topics in physics and engineering, setting the stage for the book’s main topics: physics-informed neural networks and the deep energy method. The idea of the book is to provide the basic concepts in a mathematically sound manner and yet to stay as simple as possible. To achieve this goal, mostly one-dimensional examples are investigated, such as approximating functions by neural networks or the simulation of the temperature’s evolution in a one-dimensional bar. Each chapter contains examples and exercises which are either solved analytically or in PyTorch, an open-source machine learning framework for python.
Computational Mechanics with Neural Networks
Author | : Genki Yagawa,Atsuya Oishi |
Publsiher | : Springer Nature |
Total Pages | : 233 |
Release | : 2021-02-26 |
Genre | : Technology & Engineering |
ISBN | : 9783030661113 |
Download Computational Mechanics with Neural Networks Book in PDF, Epub and Kindle
This book shows how neural networks are applied to computational mechanics. Part I presents the fundamentals of neural networks and other machine learning method in computational mechanics. Part II highlights the applications of neural networks to a variety of problems of computational mechanics. The final chapter gives perspectives to the applications of the deep learning to computational mechanics.
Computational Mechanics with Deep Learning
Author | : Genki Yagawa,Atsuya Oishi |
Publsiher | : Springer Nature |
Total Pages | : 408 |
Release | : 2022-10-31 |
Genre | : Technology & Engineering |
ISBN | : 9783031118470 |
Download Computational Mechanics with Deep Learning Book in PDF, Epub and Kindle
This book is intended for students, engineers, and researchers interested in both computational mechanics and deep learning. It presents the mathematical and computational foundations of Deep Learning with detailed mathematical formulas in an easy-to-understand manner. It also discusses various applications of Deep Learning in Computational Mechanics, with detailed explanations of the Computational Mechanics fundamentals selected there. Sample programs are included for the reader to try out in practice. This book is therefore useful for a wide range of readers interested in computational mechanics and deep learning.
Machine Learning Low Rank Approximations and Reduced Order Modeling in Computational Mechanics
Author | : Felix Fritzen,David Ryckelynck |
Publsiher | : MDPI |
Total Pages | : 254 |
Release | : 2019-09-18 |
Genre | : Technology & Engineering |
ISBN | : 9783039214099 |
Download Machine Learning Low Rank Approximations and Reduced Order Modeling in Computational Mechanics Book in PDF, Epub and Kindle
The use of machine learning in mechanics is booming. Algorithms inspired by developments in the field of artificial intelligence today cover increasingly varied fields of application. This book illustrates recent results on coupling machine learning with computational mechanics, particularly for the construction of surrogate models or reduced order models. The articles contained in this compilation were presented at the EUROMECH Colloquium 597, « Reduced Order Modeling in Mechanics of Materials », held in Bad Herrenalb, Germany, from August 28th to August 31th 2018. In this book, Artificial Neural Networks are coupled to physics-based models. The tensor format of simulation data is exploited in surrogate models or for data pruning. Various reduced order models are proposed via machine learning strategies applied to simulation data. Since reduced order models have specific approximation errors, error estimators are also proposed in this book. The proposed numerical examples are very close to engineering problems. The reader would find this book to be a useful reference in identifying progress in machine learning and reduced order modeling for computational mechanics.
Machine Learning Low Rank Approximations and Reduced Order Modeling in Computational Mechanics
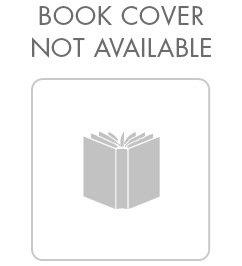
Author | : Felix Fritzen,David Ryckelynck |
Publsiher | : Unknown |
Total Pages | : 1 |
Release | : 2019 |
Genre | : Electronic books |
ISBN | : 3039214101 |
Download Machine Learning Low Rank Approximations and Reduced Order Modeling in Computational Mechanics Book in PDF, Epub and Kindle
The use of machine learning in mechanics is booming. Algorithms inspired by developments in the field of artificial intelligence today cover increasingly varied fields of application. This book illustrates recent results on coupling machine learning with computational mechanics, particularly for the construction of surrogate models or reduced order models. The articles contained in this compilation were presented at the EUROMECH Colloquium 597, « Reduced Order Modeling in Mechanics of Materials », held in Bad Herrenalb, Germany, from August 28th to August 31th 2018. In this book, Artificial Neural Networks are coupled to physics-based models. The tensor format of simulation data is exploited in surrogate models or for data pruning. Various reduced order models are proposed via machine learning strategies applied to simulation data. Since reduced order models have specific approximation errors, error estimators are also proposed in this book. The proposed numerical examples are very close to engineering problems. The reader would find this book to be a useful reference in identifying progress in machine learning and reduced order modeling for computational mechanics.
Current Trends and Open Problems in Computational Mechanics
Author | : Fadi Aldakheel,Blaž Hudobivnik,Meisam Soleimani,Henning Wessels,Christian Weißenfels,Michele Marino |
Publsiher | : Springer Nature |
Total Pages | : 587 |
Release | : 2022-03-12 |
Genre | : Science |
ISBN | : 9783030873127 |
Download Current Trends and Open Problems in Computational Mechanics Book in PDF, Epub and Kindle
This Festschrift is dedicated to Professor Dr.-Ing. habil. Peter Wriggers on the occasion of his 70th birthday. Thanks to his high dedication to research, over the years Peter Wriggers has built an international network with renowned experts in the field of computational mechanics. This is proven by the large number of contributions from friends and collaborators as well as former PhD students from all over the world. The diversity of Peter Wriggers network is mirrored by the range of topics that are covered by this book. To name only a few, these include contact mechanics, finite & virtual element technologies, micromechanics, multiscale approaches, fracture mechanics, isogeometric analysis, stochastic methods, meshfree and particle methods. Applications of numerical simulation to specific problems, e.g. Biomechanics and Additive Manufacturing is also covered. The volume intends to present an overview of the state of the art and current trends in computational mechanics for academia and industry.
Deep Learning and Physics
Author | : Akinori Tanaka,Akio Tomiya,Koji Hashimoto |
Publsiher | : Springer Nature |
Total Pages | : 207 |
Release | : 2021-03-24 |
Genre | : Science |
ISBN | : 9789813361089 |
Download Deep Learning and Physics Book in PDF, Epub and Kindle
What is deep learning for those who study physics? Is it completely different from physics? Or is it similar? In recent years, machine learning, including deep learning, has begun to be used in various physics studies. Why is that? Is knowing physics useful in machine learning? Conversely, is knowing machine learning useful in physics? This book is devoted to answers of these questions. Starting with basic ideas of physics, neural networks are derived naturally. And you can learn the concepts of deep learning through the words of physics. In fact, the foundation of machine learning can be attributed to physical concepts. Hamiltonians that determine physical systems characterize various machine learning structures. Statistical physics given by Hamiltonians defines machine learning by neural networks. Furthermore, solving inverse problems in physics through machine learning and generalization essentially provides progress and even revolutions in physics. For these reasons, in recent years interdisciplinary research in machine learning and physics has been expanding dramatically. This book is written for anyone who wants to learn, understand, and apply the relationship between deep learning/machine learning and physics. All that is needed to read this book are the basic concepts in physics: energy and Hamiltonians. The concepts of statistical mechanics and the bracket notation of quantum mechanics, which are explained in columns, are used to explain deep learning frameworks. We encourage you to explore this new active field of machine learning and physics, with this book as a map of the continent to be explored.
Machine Learning of Design Concepts
Author | : Heng Li |
Publsiher | : Computational Mechanics |
Total Pages | : 188 |
Release | : 1994 |
Genre | : Computers |
ISBN | : UOM:39015033991434 |
Download Machine Learning of Design Concepts Book in PDF, Epub and Kindle
Reviews the findings and trends of recent research on machine learning techniques and their applications in engineering design. Also presents a machine learning system that automatically generates design concepts from previous design examples. Includes abstracts of seven research papers. No index. Annotation copyright by Book News, Inc., Portland, OR