Economic Applications Of Quantile Regression
Download Economic Applications Of Quantile Regression full books in PDF, epub, and Kindle. Read online free Economic Applications Of Quantile Regression ebook anywhere anytime directly on your device. Fast Download speed and no annoying ads. We cannot guarantee that every ebooks is available!
Economic Applications of Quantile Regression
Author | : Bernd Fitzenberger,Roger Koenker,Jose A.F. Machado |
Publsiher | : Springer Science & Business Media |
Total Pages | : 325 |
Release | : 2013-03-09 |
Genre | : Business & Economics |
ISBN | : 9783662115923 |
Download Economic Applications of Quantile Regression Book in PDF, Epub and Kindle
Quantile regression has emerged as an essential statistical tool of contemporary empirical economics and biostatistics. Complementing classical least squares regression methods which are designed to estimate conditional mean models, quantile regression provides an ensemble of techniques for estimating families of conditional quantile models, thus offering a more complete view of the stochastic relationship among variables. This volume collects 12 outstanding empirical contributions in economics and offers an indispensable introduction to interpretation, implementation, and inference aspects of quantile regression.
Quantile Regression
Author | : Roger Koenker |
Publsiher | : Cambridge University Press |
Total Pages | : 367 |
Release | : 2005-05-05 |
Genre | : Business & Economics |
ISBN | : 9781139444712 |
Download Quantile Regression Book in PDF, Epub and Kindle
Quantile regression is gradually emerging as a unified statistical methodology for estimating models of conditional quantile functions. By complementing the exclusive focus of classical least squares regression on the conditional mean, quantile regression offers a systematic strategy for examining how covariates influence the location, scale and shape of the entire response distribution. This monograph is the first comprehensive treatment of the subject, encompassing models that are linear and nonlinear, parametric and nonparametric. The author has devoted more than 25 years of research to this topic. The methods in the analysis are illustrated with a variety of applications from economics, biology, ecology and finance. The treatment will find its core audiences in econometrics, statistics, and applied mathematics in addition to the disciplines cited above.
Quantile Regression for Cross Sectional and Time Series Data
Author | : Jorge M. Uribe,Montserrat Guillen |
Publsiher | : Springer Nature |
Total Pages | : 63 |
Release | : 2020-03-30 |
Genre | : Business & Economics |
ISBN | : 9783030445041 |
Download Quantile Regression for Cross Sectional and Time Series Data Book in PDF, Epub and Kindle
This brief addresses the estimation of quantile regression models from a practical perspective, which will support researchers who need to use conditional quantile regression to measure economic relationships among a set of variables. It will also benefit students using the methodology for the first time, and practitioners at private or public organizations who are interested in modeling different fragments of the conditional distribution of a given variable. The book pursues a practical approach with reference to energy markets, helping readers learn the main features of the technique more quickly. Emphasis is placed on the implementation details and the correct interpretation of the quantile regression coefficients rather than on the technicalities of the method, unlike the approach used in the majority of the literature. All applications are illustrated with R.
Handbook of Quantile Regression
Author | : Roger Koenker,Victor Chernozhukov,Xuming He,Limin Peng |
Publsiher | : CRC Press |
Total Pages | : 463 |
Release | : 2017-10-12 |
Genre | : Mathematics |
ISBN | : 9781498725293 |
Download Handbook of Quantile Regression Book in PDF, Epub and Kindle
Quantile regression constitutes an ensemble of statistical techniques intended to estimate and draw inferences about conditional quantile functions. Median regression, as introduced in the 18th century by Boscovich and Laplace, is a special case. In contrast to conventional mean regression that minimizes sums of squared residuals, median regression minimizes sums of absolute residuals; quantile regression simply replaces symmetric absolute loss by asymmetric linear loss. Since its introduction in the 1970's by Koenker and Bassett, quantile regression has been gradually extended to a wide variety of data analytic settings including time series, survival analysis, and longitudinal data. By focusing attention on local slices of the conditional distribution of response variables it is capable of providing a more complete, more nuanced view of heterogeneous covariate effects. Applications of quantile regression can now be found throughout the sciences, including astrophysics, chemistry, ecology, economics, finance, genomics, medicine, and meteorology. Software for quantile regression is now widely available in all the major statistical computing environments. The objective of this volume is to provide a comprehensive review of recent developments of quantile regression methodology illustrating its applicability in a wide range of scientific settings. The intended audience of the volume is researchers and graduate students across a diverse set of disciplines.
Quantile Regression
Author | : Cristina Davino,Marilena Furno,Domenico Vistocco |
Publsiher | : John Wiley & Sons |
Total Pages | : 288 |
Release | : 2013-12-31 |
Genre | : Mathematics |
ISBN | : 9781119975281 |
Download Quantile Regression Book in PDF, Epub and Kindle
A guide to the implementation and interpretation of Quantile Regression models This book explores the theory and numerous applications of quantile regression, offering empirical data analysis as well as the software tools to implement the methods. The main focus of this book is to provide the reader with a comprehensive description of the main issues concerning quantile regression; these include basic modeling, geometrical interpretation, estimation and inference for quantile regression, as well as issues on validity of the model, diagnostic tools. Each methodological aspect is explored and followed by applications using real data. Quantile Regression: Presents a complete treatment of quantile regression methods, including, estimation, inference issues and application of methods. Delivers a balance between methodolgy and application Offers an overview of the recent developments in the quantile regression framework and why to use quantile regression in a variety of areas such as economics, finance and computing. Features a supporting website (www.wiley.com/go/quantile_regression) hosting datasets along with R, Stata and SAS software code. Researchers and PhD students in the field of statistics, economics, econometrics, social and environmental science and chemistry will benefit from this book.
Quantile Regression
Author | : Lingxin Hao,Daniel Q. Naiman |
Publsiher | : SAGE |
Total Pages | : 142 |
Release | : 2007-04-18 |
Genre | : Business & Economics |
ISBN | : 1412926289 |
Download Quantile Regression Book in PDF, Epub and Kindle
Quantile Regression, the first book of Hao and Naiman's two-book series, establishes the seldom recognized link between inequality studies and quantile regression models. Though separate methodological literature exists for each subject, the authors seek to explore the natural connections between this increasingly sought-after tool and research topics in the social sciences. Quantile regression as a method does not rely on assumptions as restrictive as those for the classical linear regression; though more traditional models such as least squares linear regression are more widely utilized, Hao and Naiman show, in their application of quantile regression to empirical research, how this model yields a more complete understanding of inequality. Inequality is a perennial concern in the social sciences, and recently there has been much research in health inequality as well. Major software packages have also gradually implemented quantile regression. Quantile Regression will be of interest not only to the traditional social science market but other markets such as the health and public health related disciplines. Key Features: Establishes a natural link between quantile regression and inequality studies in the social sciences Contains clearly defined terms, simplified empirical equations, illustrative graphs, empirical tables and graphs from examples Includes computational codes using statistical software popular among social scientists Oriented to empirical research
Quantile Regression Under Misspecification with an Application to the U S Wage Structure
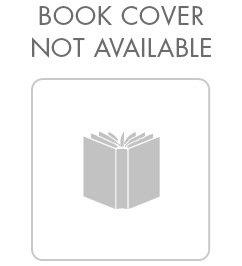
Author | : Joshua David Angrist,Victor Chernozhukov,Iván Fernández-Val |
Publsiher | : Unknown |
Total Pages | : 37 |
Release | : 2004 |
Genre | : Least squares |
ISBN | : OCLC:249905291 |
Download Quantile Regression Under Misspecification with an Application to the U S Wage Structure Book in PDF, Epub and Kindle
Quantile regression (QR) fits a linear model for conditional quantiles, just as ordinary least squares (OLS) fit a linear model for conditional means. An attractive feature of OLS is that it gives the minimum mean square error linear approximation to the conditional expectation function even when the linear model is misspecified. Empirical research using quantile regression with discrete covariates suggests that QR may have a similar property, but the exact nature of the linear approximation has remained elusive. In this paper, we show that QR can be interpreted as minimizing a weighted mean-squared error loss function for specification error. The weighting function is an average density of the dependent variable near the true conditional quantile. The weighted least squares interpretation of QR is used to derive an omitted variables bias formula and a partial quantile correlation concept, similar to the relationship between partial correlation and OLS. We also derive general asymptotic results for QR processes allowing for misspecification of the conditional quantile function, extending earlier results from a single quantile to the entire process. The approximation properties of QR are illustrated through an analysis of the wage structure and residual inequality in US census data for 1980, 1990, and 2000. The results suggest continued residual inequality growth in the 1990s, primarily in the upper half of the wage distribution and for college graduates. Keywords: residual inequality, income distribution, conditional quantiles. JEL Classifications: C13, C51, J31.
Quantile Regression for Spatial Data
Author | : Daniel P. McMillen |
Publsiher | : Springer Science & Business Media |
Total Pages | : 69 |
Release | : 2012-08-01 |
Genre | : Business & Economics |
ISBN | : 9783642318153 |
Download Quantile Regression for Spatial Data Book in PDF, Epub and Kindle
Quantile regression analysis differs from more conventional regression models in its emphasis on distributions. Whereas standard regression procedures show how the expected value of the dependent variable responds to a change in an explanatory variable, quantile regressions imply predicted changes for the entire distribution of the dependent variable. Despite its advantages, quantile regression is still not commonly used in the analysis of spatial data. The objective of this book is to make quantile regression procedures more accessible for researchers working with spatial data sets. The emphasis is on interpretation of quantile regression results. A series of examples using both simulated and actual data sets shows how readily seemingly complex quantile regression results can be interpreted with sets of well-constructed graphs. Both parametric and nonparametric versions of spatial models are considered in detail.