Large Scale Inverse Problems And Quantification Of Uncertainty
Download Large Scale Inverse Problems And Quantification Of Uncertainty full books in PDF, epub, and Kindle. Read online free Large Scale Inverse Problems And Quantification Of Uncertainty ebook anywhere anytime directly on your device. Fast Download speed and no annoying ads. We cannot guarantee that every ebooks is available!
Large Scale Inverse Problems and Quantification of Uncertainty
Author | : Lorenz Biegler,George Biros,Omar Ghattas,Matthias Heinkenschloss,David Keyes,Bani Mallick,Luis Tenorio,Bart van Bloemen Waanders,Karen Willcox,Youssef Marzouk |
Publsiher | : Wiley |
Total Pages | : 0 |
Release | : 2010-10-12 |
Genre | : Mathematics |
ISBN | : 0470685859 |
Download Large Scale Inverse Problems and Quantification of Uncertainty Book in PDF, Epub and Kindle
This book focuses on computational methods for large-scale statistical inverse problems and provides an introduction to statistical Bayesian and frequentist methodologies. Recent research advances for approximation methods are discussed, along with Kalman filtering methods and optimization-based approaches to solving inverse problems. The aim is to cross-fertilize the perspectives of researchers in the areas of data assimilation, statistics, large-scale optimization, applied and computational mathematics, high performance computing, and cutting-edge applications. The solution to large-scale inverse problems critically depends on methods to reduce computational cost. Recent research approaches tackle this challenge in a variety of different ways. Many of the computational frameworks highlighted in this book build upon state-of-the-art methods for simulation of the forward problem, such as, fast Partial Differential Equation (PDE) solvers, reduced-order models and emulators of the forward problem, stochastic spectral approximations, and ensemble-based approximations, as well as exploiting the machinery for large-scale deterministic optimization through adjoint and other sensitivity analysis methods. Key Features: • Brings together the perspectives of researchers in areas of inverse problems and data assimilation. • Assesses the current state-of-the-art and identify needs and opportunities for future research. • Focuses on the computational methods used to analyze and simulate inverse problems. • Written by leading experts of inverse problems and uncertainty quantification. Graduate students and researchers working in statistics, mathematics and engineering will benefit from this book.
Large Scale Inverse Problems and Quantification of Uncertainty
Author | : Lorenz Biegler,George Biros,Omar Ghattas,Matthias Heinkenschloss,David Keyes,Bani Mallick,Luis Tenorio,Bart van Bloemen Waanders,Karen Willcox,Youssef Marzouk |
Publsiher | : John Wiley & Sons |
Total Pages | : 403 |
Release | : 2011-06-24 |
Genre | : Mathematics |
ISBN | : 9781119957584 |
Download Large Scale Inverse Problems and Quantification of Uncertainty Book in PDF, Epub and Kindle
This book focuses on computational methods for large-scale statistical inverse problems and provides an introduction to statistical Bayesian and frequentist methodologies. Recent research advances for approximation methods are discussed, along with Kalman filtering methods and optimization-based approaches to solving inverse problems. The aim is to cross-fertilize the perspectives of researchers in the areas of data assimilation, statistics, large-scale optimization, applied and computational mathematics, high performance computing, and cutting-edge applications. The solution to large-scale inverse problems critically depends on methods to reduce computational cost. Recent research approaches tackle this challenge in a variety of different ways. Many of the computational frameworks highlighted in this book build upon state-of-the-art methods for simulation of the forward problem, such as, fast Partial Differential Equation (PDE) solvers, reduced-order models and emulators of the forward problem, stochastic spectral approximations, and ensemble-based approximations, as well as exploiting the machinery for large-scale deterministic optimization through adjoint and other sensitivity analysis methods. Key Features: Brings together the perspectives of researchers in areas of inverse problems and data assimilation. Assesses the current state-of-the-art and identify needs and opportunities for future research. Focuses on the computational methods used to analyze and simulate inverse problems. Written by leading experts of inverse problems and uncertainty quantification. Graduate students and researchers working in statistics, mathematics and engineering will benefit from this book.
Computational Uncertainty Quantification for Inverse Problems
Author | : Johnathan M. Bardsley |
Publsiher | : SIAM |
Total Pages | : 135 |
Release | : 2018-08-01 |
Genre | : Science |
ISBN | : 9781611975383 |
Download Computational Uncertainty Quantification for Inverse Problems Book in PDF, Epub and Kindle
This book is an introduction to both computational inverse problems and uncertainty quantification (UQ) for inverse problems. The book also presents more advanced material on Bayesian methods and UQ, including Markov chain Monte Carlo sampling methods for UQ in inverse problems. Each chapter contains MATLAB® code that implements the algorithms and generates the figures, as well as a large number of exercises accessible to both graduate students and researchers. Computational Uncertainty Quantification for Inverse Problems is intended for graduate students, researchers, and applied scientists. It is appropriate for courses on computational inverse problems, Bayesian methods for inverse problems, and UQ methods for inverse problems.
An Introduction to Data Analysis and Uncertainty Quantification for Inverse Problems
Author | : Luis Tenorio |
Publsiher | : SIAM |
Total Pages | : 275 |
Release | : 2017-07-06 |
Genre | : Mathematics |
ISBN | : 9781611974911 |
Download An Introduction to Data Analysis and Uncertainty Quantification for Inverse Problems Book in PDF, Epub and Kindle
Inverse problems are found in many applications, such as medical imaging, engineering, astronomy, and geophysics, among others. To solve an inverse problem is to recover an object from noisy, usually indirect observations. Solutions to inverse problems are subject to many potential sources of error introduced by approximate mathematical models, regularization methods, numerical approximations for efficient computations, noisy data, and limitations in the number of observations; thus it is important to include an assessment of the uncertainties as part of the solution. Such assessment is interdisciplinary by nature, as it requires, in addition to knowledge of the particular application, methods from applied mathematics, probability, and statistics. This book bridges applied mathematics and statistics by providing a basic introduction to probability and statistics for uncertainty quantification in the context of inverse problems, as well as an introduction to statistical regularization of inverse problems. The author covers basic statistical inference, introduces the framework of ill-posed inverse problems, and explains statistical questions that arise in their applications. An Introduction to Data Analysis and Uncertainty Quantification for Inverse Problems?includes many examples that explain techniques which are useful to address general problems arising in uncertainty quantification, Bayesian and non-Bayesian statistical methods and discussions of their complementary roles, and analysis of a real data set to illustrate the methodology covered throughout the book.
An Introduction to Data Analysis and Uncertainty Quantification for Inverse Problems
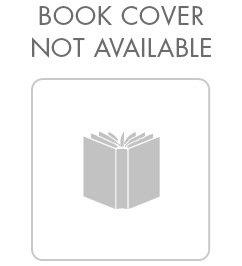
Author | : Luis Tenorio |
Publsiher | : Unknown |
Total Pages | : 269 |
Release | : 2017 |
Genre | : Electronic books |
ISBN | : LCCN:2017016391 |
Download An Introduction to Data Analysis and Uncertainty Quantification for Inverse Problems Book in PDF, Epub and Kindle
Abstract: Inverse problems are found in many applications, such as medical imaging, engineering, astronomy, and geophysics, among others. To solve an inverse problem is to recover an object from noisy, usually indirect observations. Solutions to inverse problems are subject to many potential sources of error introduced by approximate mathematical models, regularization methods, numerical approximations for efficient computations, noisy data, and limitations in the number of observations; thus it is important to include an assessment of the uncertainties as part of the solution. Such assessment is interdisciplinary by nature, as it requires, in addition to knowledge of the particular application, methods from applied mathematics, probability, and statistics. This book bridges applied mathematics and statistics by providing a basic introduction to probability and statistics for uncertainty quantification in the context of inverse problems, as well as an introduction to statistical regularization of inverse problems. The author covers basic statistical inference, introduces the framework of ill-posed inverse problems, and explains statistical questions that arise in their applications. An Introduction to Data Analysis and Uncertainty Quantification for Inverse Problems includes many examples that explain techniques which are useful to address general problems arising in uncertainty quantification, Bayesian and non-Bayesian statistical methods and discussions of their complementary roles, and analysis of a real data set to illustrate the methodology covered throughout the book
Handbook of Dynamic Data Driven Applications Systems
Author | : Frederica Darema,Erik P. Blasch,Sai Ravela,Alex J. Aved |
Publsiher | : Springer Nature |
Total Pages | : 937 |
Release | : 2023-10-16 |
Genre | : Computers |
ISBN | : 9783031279867 |
Download Handbook of Dynamic Data Driven Applications Systems Book in PDF, Epub and Kindle
This Second Volume in the series Handbook of Dynamic Data Driven Applications Systems (DDDAS) expands the scope of the methods and the application areas presented in the first Volume and aims to provide additional and extended content of the increasing set of science and engineering advances for new capabilities enabled through DDDAS. The methods and examples of breakthroughs presented in the book series capture the DDDAS paradigm and its scientific and technological impact and benefits. The DDDAS paradigm and the ensuing DDDAS-based frameworks for systems’ analysis and design have been shown to engender new and advanced capabilities for understanding, analysis, and management of engineered, natural, and societal systems (“applications systems”), and for the commensurate wide set of scientific and engineering fields and applications, as well as foundational areas. The DDDAS book series aims to be a reference source of many of the important research and development efforts conducted under the rubric of DDDAS, and to also inspire the broader communities of researchers and developers about the potential in their respective areas of interest, of the application and the exploitation of the DDDAS paradigm and the ensuing frameworks, through the examples and case studies presented, either within their own field or other fields of study. As in the first volume, the chapters in this book reflect research work conducted over the years starting in the 1990’s to the present. Here, the theory and application content are considered for: Foundational Methods Materials Systems Structural Systems Energy Systems Environmental Systems: Domain Assessment & Adverse Conditions/Wildfires Surveillance Systems Space Awareness Systems Healthcare Systems Decision Support Systems Cyber Security Systems Design of Computer Systems The readers of this book series will benefit from DDDAS theory advances such as object estimation, information fusion, and sensor management. The increased interest in Artificial Intelligence (AI), Machine Learning and Neural Networks (NN) provides opportunities for DDDAS-based methods to show the key role DDDAS plays in enabling AI capabilities; address challenges that ML-alone does not, and also show how ML in combination with DDDAS-based methods can deliver the advanced capabilities sought; likewise, infusion of DDDAS-like approaches in NN-methods strengthens such methods. Moreover, the “DDDAS-based Digital Twin” or “Dynamic Digital Twin”, goes beyond the traditional DT notion where the model and the physical system are viewed side-by-side in a static way, to a paradigm where the model dynamically interacts with the physical system through its instrumentation, (per the DDDAS feed-back control loop between model and instrumentation).
Handbook of Mathematical Methods in Imaging
Author | : Otmar Scherzer |
Publsiher | : Springer Science & Business Media |
Total Pages | : 1626 |
Release | : 2010-11-23 |
Genre | : Mathematics |
ISBN | : 9780387929194 |
Download Handbook of Mathematical Methods in Imaging Book in PDF, Epub and Kindle
The Handbook of Mathematical Methods in Imaging provides a comprehensive treatment of the mathematical techniques used in imaging science. The material is grouped into two central themes, namely, Inverse Problems (Algorithmic Reconstruction) and Signal and Image Processing. Each section within the themes covers applications (modeling), mathematics, numerical methods (using a case example) and open questions. Written by experts in the area, the presentation is mathematically rigorous. The entries are cross-referenced for easy navigation through connected topics. Available in both print and electronic forms, the handbook is enhanced by more than 150 illustrations and an extended bibliography. It will benefit students, scientists and researchers in applied mathematics. Engineers and computer scientists working in imaging will also find this handbook useful.
Numerical Analysis and Optimization
Author | : Mehiddin Al-Baali,Lucio Grandinetti,Anton Purnama |
Publsiher | : Springer |
Total Pages | : 344 |
Release | : 2015-07-16 |
Genre | : Mathematics |
ISBN | : 9783319176895 |
Download Numerical Analysis and Optimization Book in PDF, Epub and Kindle
Presenting the latest findings in the field of numerical analysis and optimization, this volume balances pure research with practical applications of the subject. Accompanied by detailed tables, figures, and examinations of useful software tools, this volume will equip the reader to perform detailed and layered analysis of complex datasets. Many real-world complex problems can be formulated as optimization tasks. Such problems can be characterized as large scale, unconstrained, constrained, non-convex, non-differentiable, and discontinuous, and therefore require adequate computational methods, algorithms, and software tools. These same tools are often employed by researchers working in current IT hot topics such as big data, optimization and other complex numerical algorithms on the cloud, devising special techniques for supercomputing systems. The list of topics covered include, but are not limited to: numerical analysis, numerical optimization, numerical linear algebra, numerical differential equations, optimal control, approximation theory, applied mathematics, algorithms and software developments, derivative free optimization methods and programming models. The volume also examines challenging applications to various types of computational optimization methods which usually occur in statistics, econometrics, finance, physics, medicine, biology, engineering and industrial sciences.