Machine Learning Solutions
Download Machine Learning Solutions full books in PDF, epub, and Kindle. Read online free Machine Learning Solutions ebook anywhere anytime directly on your device. Fast Download speed and no annoying ads. We cannot guarantee that every ebooks is available!
Mathematics for Machine Learning
Author | : Marc Peter Deisenroth,A. Aldo Faisal,Cheng Soon Ong |
Publsiher | : Cambridge University Press |
Total Pages | : 391 |
Release | : 2020-04-23 |
Genre | : Computers |
ISBN | : 9781108470049 |
Download Mathematics for Machine Learning Book in PDF, Epub and Kindle
Distills key concepts from linear algebra, geometry, matrices, calculus, optimization, probability and statistics that are used in machine learning.
Machine Learning Solutions
Author | : Jalaj Thanaki |
Publsiher | : Packt Publishing Ltd |
Total Pages | : 567 |
Release | : 2018-04-27 |
Genre | : Computers |
ISBN | : 9781788398893 |
Download Machine Learning Solutions Book in PDF, Epub and Kindle
Practical, hands-on solutions in Python to overcome any problem in Machine Learning Key Features Master the advanced concepts, methodologies, and use cases of machine learning Build ML applications for analytics, NLP and computer vision domains Solve the most common problems in building machine learning models Book Description Machine learning (ML) helps you find hidden insights from your data without the need for explicit programming. This book is your key to solving any kind of ML problem you might come across in your job. You’ll encounter a set of simple to complex problems while building ML models, and you'll not only resolve these problems, but you’ll also learn how to build projects based on each problem, with a practical approach and easy-to-follow examples. The book includes a wide range of applications: from analytics and NLP, to computer vision domains. Some of the applications you will be working on include stock price prediction, a recommendation engine, building a chat-bot, a facial expression recognition system, and many more. The problem examples we cover include identifying the right algorithm for your dataset and use cases, creating and labeling datasets, getting enough clean data to carry out processing, identifying outliers, overftting datasets, hyperparameter tuning, and more. Here, you'll also learn to make more timely and accurate predictions. In addition, you'll deal with more advanced use cases, such as building a gaming bot, building an extractive summarization tool for medical documents, and you'll also tackle the problems faced while building an ML model. By the end of this book, you'll be able to fine-tune your models as per your needs to deliver maximum productivity. What you will learn Select the right algorithm to derive the best solution in ML domains Perform predictive analysis effciently using ML algorithms Predict stock prices using the stock index value Perform customer analytics for an e-commerce platform Build recommendation engines for various domains Build NLP applications for the health domain Build language generation applications using different NLP techniques Build computer vision applications such as facial emotion recognition Who this book is for This book is for the intermediate users such as machine learning engineers, data engineers, data scientists, and more, who want to solve simple to complex machine learning problems in their day-to-day work and build powerful and efficient machine learning models. A basic understanding of the machine learning concepts and some experience with Python programming is all you need to get started with this book.
Pattern Recognition and Machine Learning
Author | : Christopher M. Bishop |
Publsiher | : Springer |
Total Pages | : 0 |
Release | : 2016-08-23 |
Genre | : Computers |
ISBN | : 1493938436 |
Download Pattern Recognition and Machine Learning Book in PDF, Epub and Kindle
This is the first textbook on pattern recognition to present the Bayesian viewpoint. The book presents approximate inference algorithms that permit fast approximate answers in situations where exact answers are not feasible. It uses graphical models to describe probability distributions when no other books apply graphical models to machine learning. No previous knowledge of pattern recognition or machine learning concepts is assumed. Familiarity with multivariate calculus and basic linear algebra is required, and some experience in the use of probabilities would be helpful though not essential as the book includes a self-contained introduction to basic probability theory.
Fundamentals of Machine Learning for Predictive Data Analytics second edition
Author | : John D. Kelleher,Brian Mac Namee,Aoife D'Arcy |
Publsiher | : MIT Press |
Total Pages | : 853 |
Release | : 2020-10-20 |
Genre | : Computers |
ISBN | : 9780262361101 |
Download Fundamentals of Machine Learning for Predictive Data Analytics second edition Book in PDF, Epub and Kindle
The second edition of a comprehensive introduction to machine learning approaches used in predictive data analytics, covering both theory and practice. Machine learning is often used to build predictive models by extracting patterns from large datasets. These models are used in predictive data analytics applications including price prediction, risk assessment, predicting customer behavior, and document classification. This introductory textbook offers a detailed and focused treatment of the most important machine learning approaches used in predictive data analytics, covering both theoretical concepts and practical applications. Technical and mathematical material is augmented with explanatory worked examples, and case studies illustrate the application of these models in the broader business context. This second edition covers recent developments in machine learning, especially in a new chapter on deep learning, and two new chapters that go beyond predictive analytics to cover unsupervised learning and reinforcement learning.
The Machine Learning Solutions Architect Handbook
Author | : David Ping |
Publsiher | : Packt Publishing Ltd |
Total Pages | : 442 |
Release | : 2022-01-21 |
Genre | : Computers |
ISBN | : 9781801070416 |
Download The Machine Learning Solutions Architect Handbook Book in PDF, Epub and Kindle
Build highly secure and scalable machine learning platforms to support the fast-paced adoption of machine learning solutions Key Features Explore different ML tools and frameworks to solve large-scale machine learning challenges in the cloud Build an efficient data science environment for data exploration, model building, and model training Learn how to implement bias detection, privacy, and explainability in ML model development Book DescriptionWhen equipped with a highly scalable machine learning (ML) platform, organizations can quickly scale the delivery of ML products for faster business value realization. There is a huge demand for skilled ML solutions architects in different industries, and this handbook will help you master the design patterns, architectural considerations, and the latest technology insights you’ll need to become one. You’ll start by understanding ML fundamentals and how ML can be applied to solve real-world business problems. Once you've explored a few leading problem-solving ML algorithms, this book will help you tackle data management and get the most out of ML libraries such as TensorFlow and PyTorch. Using open source technology such as Kubernetes/Kubeflow to build a data science environment and ML pipelines will be covered next, before moving on to building an enterprise ML architecture using Amazon Web Services (AWS). You’ll also learn about security and governance considerations, advanced ML engineering techniques, and how to apply bias detection, explainability, and privacy in ML model development. By the end of this book, you’ll be able to design and build an ML platform to support common use cases and architecture patterns like a true professional. What you will learn Apply ML methodologies to solve business problems Design a practical enterprise ML platform architecture Implement MLOps for ML workflow automation Build an end-to-end data management architecture using AWS Train large-scale ML models and optimize model inference latency Create a business application using an AI service and a custom ML model Use AWS services to detect data and model bias and explain models Who this book is for This book is for data scientists, data engineers, cloud architects, and machine learning enthusiasts who want to become machine learning solutions architects. You’ll need basic knowledge of the Python programming language, AWS, linear algebra, probability, and networking concepts before you get started with this handbook.
Applied Machine Learning Solutions with Python
Author | : Siddhanta Bhatta |
Publsiher | : BPB Publications |
Total Pages | : 418 |
Release | : 2021-08-31 |
Genre | : Computers |
ISBN | : 9789391030438 |
Download Applied Machine Learning Solutions with Python Book in PDF, Epub and Kindle
A problem-focused guide for tackling industrial machine learning issues with methods and frameworks chosen by experts. KEY FEATURES ● Popular techniques for problem formulation, data collection, and data cleaning in machine learning. ● Comprehensive and useful machine learning tools such as MLFlow, Streamlit, and many more. ● Covers numerous machine learning libraries, including Tensorflow, FastAI, Scikit-Learn, Pandas, and Numpy. DESCRIPTION This book discusses how to apply machine learning to real-world problems by utilizing real-world data. In this book, you will investigate data sources, become acquainted with data pipelines, and practice how machine learning works through numerous examples and case studies. The book begins with high-level concepts and implementation (with code!) and progresses towards the real-world of ML systems. It briefly discusses various concepts of Statistics and Linear Algebra. You will learn how to formulate a problem, collect data, build a model, and tune it. You will learn about use cases for data analytics, computer vision, and natural language processing. You will also explore nonlinear architecture, thus enabling you to build models with multiple inputs and outputs. You will get trained on creating a machine learning profile, various machine learning libraries, Statistics, and FAST API. Throughout the book, you will use Python to experiment with machine learning libraries such as Tensorflow, Scikit-learn, Spacy, and FastAI. The book will help train our models on both Kaggle and our datasets. WHAT YOU WILL LEARN ● Construct a machine learning problem, evaluate the feasibility, and gather and clean data. ● Learn to explore data first, select, and train machine learning models. ● Fine-tune the chosen model, deploy, and monitor it in production. ● Discover popular models for data analytics, computer vision, and Natural Language Processing. ● Create a machine learning profile and contribute to the community. WHO THIS BOOK IS FOR This book caters to beginners in machine learning, software engineers, and students who want to gain a good understanding of machine learning concepts and create production-ready ML systems. This book assumes you have a beginner-level understanding of Python. TABLE OF CONTENTS 1. Introduction to Machine Learning 2. Problem Formulation in Machine Learning 3. Data Acquisition and Cleaning 4. Exploratory Data Analysis 5. Model Building and Tuning 6. Taking Our Model into Production 7. Data Analytics Use Case 8. Building a Custom Image Classifier from Scratch 9. Building a News Summarization App Using Transformers 10. Multiple Inputs and Multiple Output Models 11. Contributing to the Community 12. Creating Your Project 13. Crash Course in Numpy, Matplotlib, and Pandas 14. Crash Course in Linear Algebra and Statistics 15. Crash Course in FastAPI
Optimizing AI and Machine Learning Solutions
Author | : Mirza Rahim Baig |
Publsiher | : BPB Publications |
Total Pages | : 477 |
Release | : 2024-03-04 |
Genre | : Computers |
ISBN | : 9789355519818 |
Download Optimizing AI and Machine Learning Solutions Book in PDF, Epub and Kindle
Build high-impact ML/AI solutions by optimizing each step KEY FEATURES ● Build and fine-tune models for maximum performance. ● Practical tips to make your own state-of-the-art AI/ML models. ● ML/AI problem solving tips with multiple case studies to tackle real-world challenges. DESCRIPTION This book approaches data science solution building using a principled framework and case studies with extensive hands-on guidance. It will teach the readers optimization at each step, whether it is problem formulation or hyperparameter tuning for deep learning models. This book keeps the reader pragmatic and guides them toward practical solutions by discussing the essential ML concepts, including problem formulation, data preparation, and evaluation techniques. Further, the reader will be able to learn how to apply model optimization with advanced algorithms, hyperparameter tuning, and strategies against overfitting. They will also benefit from deep learning by optimizing models for image processing, natural language processing, and specialized applications. The reader can put theory into practice with hands-on case studies and code examples, reinforcing their understanding. With this book, the reader will be able to create high-impact, high-value ML/AI solutions by optimizing each step of the solution building process, which is the ultimate goal of every data science professional. WHAT YOU WILL LEARN ● End-to-end solutions to ML/AI problems. ● Data augmentation and transfer learning. ● Optimizing AI/ML solutions at each step of development. ● Multiple hands-on real case studies. ● Choose between various ML/AI models. WHO THIS BOOK IS FOR This book empowers data scientists, developers, and AI enthusiasts at all levels to unlock the full potential of their ML solutions. This guide equips you to become a confident AI optimization expert. TABLE OF CONTENTS 1. Optimizing a Machine Learning /Artificial Intelligence Solution 2. ML Problem Formulation: Setting the Right Objective 3. Data Collection and Pre-processing 4. Model Evaluation and Debugging 5. Imbalanced Machine Learning 6. Hyper-parameter Tuning 7. Parameter Optimization Algorithms 8. Optimizing Deep Learning Models 9. Optimizing Image Models 10. Optimizing Natural Language Processing Models 11. Transfer Learning
Machine Learning Solutions
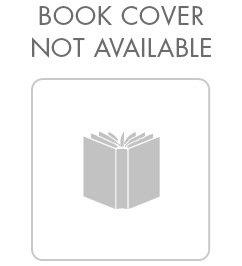
Author | : Jalaj Thanaki |
Publsiher | : Unknown |
Total Pages | : 566 |
Release | : 2018 |
Genre | : Software engineering |
ISBN | : OCLC:1105789361 |
Download Machine Learning Solutions Book in PDF, Epub and Kindle
Practical, hands-on solutions in Python to overcome any problem in Machine Learning About This Book Master the advanced concepts, methodologies, and use cases of machine learning Build ML applications for analytics, NLP and computer vision domains Solve the most common problems in building machine learning models Who This Book Is For This book is for the intermediate users such as machine learning engineers, data engineers, data scientists, and more, who want to solve simple to complex machine learning problems in their day-to-day work and build powerful and efficient machine learning models. A basic understanding of the machine learning concepts and some experience with Python programming is all you need to get started with this book. What You Will Learn Select the right algorithm to derive the best solution in ML domains Perform predictive analysis effciently using ML algorithms Predict stock prices using the stock index value Perform customer analytics for an e-commerce platform Build recommendation engines for various domains Build NLP applications for the health domain Build language generation applications using different NLP techniques Build computer vision applications such as facial emotion recognition In Detail Machine learning (ML) helps you find hidden insights from your data without the need for explicit programming. This book is your key to solving any kind of ML problem you might come across in your job. You'll encounter a set of simple to complex problems while building ML models, and you'll not only resolve these problems, but you'll also learn how to build projects based on each problem, with a practical approach and easy-to-follow examples. The book includes a wide range of applications: from analytics and NLP, to computer vision domains. Some of the applications you will be working on include stock price prediction, a recommendation engine, building a chat-bot, a facial expression recognition system, and many more. The problem examples we cover include identifying the right algorithm for your dataset and use cases, creating and labeling datasets, getting enough clean data to carry out processing, identifying outliers, overftting datasets, hyperparameter tuning, and more. Here, you'll also learn to make more timely and accurate predictions. In addition, you'll deal with more advanced use cases, such as building a gaming bot, building an extractive summarization tool for medical documents, and you'll also tackle the problems ...