Multi Omic Data Integration
Download Multi Omic Data Integration full books in PDF, epub, and Kindle. Read online free Multi Omic Data Integration ebook anywhere anytime directly on your device. Fast Download speed and no annoying ads. We cannot guarantee that every ebooks is available!
Multi omic Data Integration
Author | : Paolo Tieri,Christine Nardini,Jennifer Elizabeth Dent |
Publsiher | : Frontiers Media SA |
Total Pages | : 137 |
Release | : 2015-09-17 |
Genre | : Electronic book |
ISBN | : 9782889196487 |
Download Multi omic Data Integration Book in PDF, Epub and Kindle
Stable, predictive biomarkers and interpretable disease signatures are seen as a significant step towards personalized medicine. In this perspective, integration of multi-omic data coming from genomics, transcriptomics, glycomics, proteomics, metabolomics is a powerful strategy to reconstruct and analyse complex multi-dimensional interactions, enabling deeper mechanistic and medical insight. At the same time, there is a rising concern that much of such different omic data –although often publicly and freely available- lie in databases and repositories underutilised or not used at all. Issues coming from lack of standardisation and shared biological identities are also well-known. From these considerations, a novel, pressing request arises from the life sciences to design methodologies and approaches that allow for these data to be interpreted as a whole, i.e. as intertwined molecular signatures containing genes, proteins, mRNAs and miRNAs, able to capture inter-layers connections and complexity. Papers discuss data integration approaches and methods of several types and extents, their application in understanding the pathogenesis of specific diseases or in identifying candidate biomarkers to exploit the full benefit of multi-omic datasets and their intrinsic information content. Topics of interest include, but are not limited to: • Methods for the integration of layered data, including, but not limited to, genomics, transcriptomics, glycomics, proteomics, metabolomics; • Application of multi-omic data integration approaches for diagnostic biomarker discovery in any field of the life sciences; • Innovative approaches for the analysis and the visualization of multi-omic datasets; • Methods and applications for systematic measurements from single/undivided samples (comprising genomic, transcriptomic, proteomic, metabolomic measurements, among others); • Multi-scale approaches for integrated dynamic modelling and simulation; • Implementation of applications, computational resources and repositories devoted to data integration including, but not limited to, data warehousing, database federation, semantic integration, service-oriented and/or wiki integration; • Issues related to the definition and implementation of standards, shared identities and semantics, with particular focus on the integration problem. Research papers, reviews and short communications on all topics related to the above issues were welcomed.
Integrating Omics Data
Author | : George Tseng,Debashis Ghosh,Xianghong Jasmine Zhou |
Publsiher | : Cambridge University Press |
Total Pages | : 497 |
Release | : 2015-09-23 |
Genre | : Mathematics |
ISBN | : 9781107069114 |
Download Integrating Omics Data Book in PDF, Epub and Kindle
Tutorial chapters by leaders in the field introduce state-of-the-art methods to handle information integration problems of omics data.
Computational Genomics with R
Author | : Altuna Akalin |
Publsiher | : CRC Press |
Total Pages | : 462 |
Release | : 2020-12-16 |
Genre | : Mathematics |
ISBN | : 9781498781862 |
Download Computational Genomics with R Book in PDF, Epub and Kindle
Computational Genomics with R provides a starting point for beginners in genomic data analysis and also guides more advanced practitioners to sophisticated data analysis techniques in genomics. The book covers topics from R programming, to machine learning and statistics, to the latest genomic data analysis techniques. The text provides accessible information and explanations, always with the genomics context in the background. This also contains practical and well-documented examples in R so readers can analyze their data by simply reusing the code presented. As the field of computational genomics is interdisciplinary, it requires different starting points for people with different backgrounds. For example, a biologist might skip sections on basic genome biology and start with R programming, whereas a computer scientist might want to start with genome biology. After reading: You will have the basics of R and be able to dive right into specialized uses of R for computational genomics such as using Bioconductor packages. You will be familiar with statistics, supervised and unsupervised learning techniques that are important in data modeling, and exploratory analysis of high-dimensional data. You will understand genomic intervals and operations on them that are used for tasks such as aligned read counting and genomic feature annotation. You will know the basics of processing and quality checking high-throughput sequencing data. You will be able to do sequence analysis, such as calculating GC content for parts of a genome or finding transcription factor binding sites. You will know about visualization techniques used in genomics, such as heatmaps, meta-gene plots, and genomic track visualization. You will be familiar with analysis of different high-throughput sequencing data sets, such as RNA-seq, ChIP-seq, and BS-seq. You will know basic techniques for integrating and interpreting multi-omics datasets. Altuna Akalin is a group leader and head of the Bioinformatics and Omics Data Science Platform at the Berlin Institute of Medical Systems Biology, Max Delbrück Center, Berlin. He has been developing computational methods for analyzing and integrating large-scale genomics data sets since 2002. He has published an extensive body of work in this area. The framework for this book grew out of the yearly computational genomics courses he has been organizing and teaching since 2015.
Multivariate Data Integration Using R
Author | : Kim-Anh Lê Cao,Zoe Marie Welham |
Publsiher | : CRC Press |
Total Pages | : 316 |
Release | : 2021-11-08 |
Genre | : Computers |
ISBN | : 9781000472196 |
Download Multivariate Data Integration Using R Book in PDF, Epub and Kindle
Large biological data, which are often noisy and high-dimensional, have become increasingly prevalent in biology and medicine. There is a real need for good training in statistics, from data exploration through to analysis and interpretation. This book provides an overview of statistical and dimension reduction methods for high-throughput biological data, with a specific focus on data integration. It starts with some biological background, key concepts underlying the multivariate methods, and then covers an array of methods implemented using the mixOmics package in R. Features: Provides a broad and accessible overview of methods for multi-omics data integration Covers a wide range of multivariate methods, each designed to answer specific biological questions Includes comprehensive visualisation techniques to aid in data interpretation Includes many worked examples and case studies using real data Includes reproducible R code for each multivariate method, using the mixOmics package The book is suitable for researchers from a wide range of scientific disciplines wishing to apply these methods to obtain new and deeper insights into biological mechanisms and biomedical problems. The suite of tools introduced in this book will enable students and scientists to work at the interface between, and provide critical collaborative expertise to, biologists, bioinformaticians, statisticians and clinicians.
Metagenomics of the Human Body
Author | : Karen E. Nelson |
Publsiher | : Springer Science & Business Media |
Total Pages | : 302 |
Release | : 2010-11-16 |
Genre | : Science |
ISBN | : 9781441970893 |
Download Metagenomics of the Human Body Book in PDF, Epub and Kindle
The book brings a completely different perspective than available books by combining the information gained from the human genome with that derived from parallel metagenomic studies, and new results from investigating the effects of these microbes on the host immune system. Although there are a number of books that focus on the human genome that are currently available, there are no books that bring to the forefront the mix of the human genome and the genomes and metagenomes of the microbial species that live within and on us.
Multi omic Data Integration in Oncology
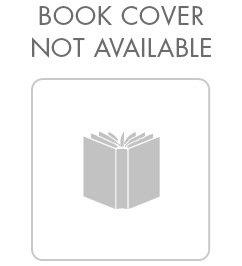
Author | : Chiara Romualdi,Enrica Calura,Davide Risso,Sampsa Hautaniemi,Francesca Finotello |
Publsiher | : Unknown |
Total Pages | : 0 |
Release | : 2020 |
Genre | : Electronic Book |
ISBN | : OCLC:1368448508 |
Download Multi omic Data Integration in Oncology Book in PDF, Epub and Kindle
This eBook is a collection of articles from a Frontiers Research Topic. Frontiers Research Topics are very popular trademarks of the Frontiers Journals Series: they are collections of at least ten articles, all centered on a particular subject. With their unique mix of varied contributions from Original Research to Review Articles, Frontiers Research Topics unify the most influential researchers, the latest key findings and historical advances in a hot research area! Find out more on how to host your own Frontiers Research Topic or contribute to one as an author by contacting the Frontiers Editorial Office: frontiersin.org/about/contact.
Multi omic Data Integration in Oncology
Author | : Chiara Romualdi,Enrica Calura,Davide Risso,Sampsa Hautaniemi,Francesca Finotello |
Publsiher | : Frontiers Media SA |
Total Pages | : 187 |
Release | : 2020-12-03 |
Genre | : Medical |
ISBN | : 9782889661510 |
Download Multi omic Data Integration in Oncology Book in PDF, Epub and Kindle
This eBook is a collection of articles from a Frontiers Research Topic. Frontiers Research Topics are very popular trademarks of the Frontiers Journals Series: they are collections of at least ten articles, all centered on a particular subject. With their unique mix of varied contributions from Original Research to Review Articles, Frontiers Research Topics unify the most influential researchers, the latest key findings and historical advances in a hot research area! Find out more on how to host your own Frontiers Research Topic or contribute to one as an author by contacting the Frontiers Editorial Office: frontiersin.org/about/contact.
Machine Learning Methods for Multi Omics Data Integration
Author | : Abedalrhman Alkhateeb,Luis Rueda |
Publsiher | : Springer Nature |
Total Pages | : 171 |
Release | : 2023-12-15 |
Genre | : Science |
ISBN | : 9783031365027 |
Download Machine Learning Methods for Multi Omics Data Integration Book in PDF, Epub and Kindle
The advancement of biomedical engineering has enabled the generation of multi-omics data by developing high-throughput technologies, such as next-generation sequencing, mass spectrometry, and microarrays. Large-scale data sets for multiple omics platforms, including genomics, transcriptomics, proteomics, and metabolomics, have become more accessible and cost-effective over time. Integrating multi-omics data has become increasingly important in many research fields, such as bioinformatics, genomics, and systems biology. This integration allows researchers to understand complex interactions between biological molecules and pathways. It enables us to comprehensively understand complex biological systems, leading to new insights into disease mechanisms, drug discovery, and personalized medicine. Still, integrating various heterogeneous data types into a single learning model also comes with challenges. In this regard, learning algorithms have been vital in analyzing and integrating these large-scale heterogeneous data sets into one learning model. This book overviews the latest multi-omics technologies, machine learning techniques for data integration, and multi-omics databases for validation. It covers different types of learning for supervised and unsupervised learning techniques, including standard classifiers, deep learning, tensor factorization, ensemble learning, and clustering, among others. The book categorizes different levels of integrations, ranging from early, middle, or late-stage among multi-view models. The underlying models target different objectives, such as knowledge discovery, pattern recognition, disease-related biomarkers, and validation tools for multi-omics data. Finally, the book emphasizes practical applications and case studies, making it an essential resource for researchers and practitioners looking to apply machine learning to their multi-omics data sets. The book covers data preprocessing, feature selection, and model evaluation, providing readers with a practical guide to implementing machine learning techniques on various multi-omics data sets.