Towards Useful Ai Interpretability For Humans Via Interactive Ai Explanations
Download Towards Useful Ai Interpretability For Humans Via Interactive Ai Explanations full books in PDF, epub, and Kindle. Read online free Towards Useful Ai Interpretability For Humans Via Interactive Ai Explanations ebook anywhere anytime directly on your device. Fast Download speed and no annoying ads. We cannot guarantee that every ebooks is available!
Towards Useful AI Interpretability for Humans Via Interactive AI Explanations
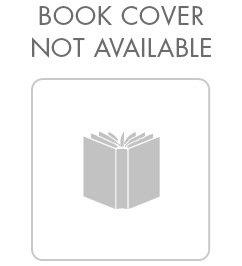
Author | : Hua Shen |
Publsiher | : Unknown |
Total Pages | : 0 |
Release | : 2023 |
Genre | : Electronic Book |
ISBN | : OCLC:1424639332 |
Download Towards Useful AI Interpretability for Humans Via Interactive AI Explanations Book in PDF, Epub and Kindle
Advancements in deep learning have revolutionized AI systems, enabling collaboration between humans and AI to enhance performance in specific tasks. AI explanations play a crucial role in aiding human understanding, control, and improvement of AI systems regarding various criteria such as fairness, safety, and trustworthiness. Despite the proliferation of eXplainable AI (XAI) approaches, the practical usefulness of AI explanations in human-AI collaborative systems remains underexplored. This doctoral research aims to evaluate and enhance the usefulness of AI explanations for humans in practical human-AI collaboration. I break down the research goal of investigating and improving human-centered useful AI explanations into three research questions: RQ1: Are cutting-edge AI explanations useful for humans in practice (Part I)? RQ2: What's the disparity between AI explanations and practical user demands (Part II)? RQ3: How to empower useful AI explanations with human-AI interaction (Part III)? We examined the three research questions by conducting four projects. To answer RQ1, we deployed two real-world human evaluation studies on analyzing computer vision AI model errors with post-hoc explanations and simulating NLP AI model predictions with inherent explanations, respectively. The two studies unveil that, surprisingly, AI explanations are not always useful for humans to analyze AI predictions in practice. This motivates our research for RQ2 -- gaining insights into disparities between the status quo of AI explanations and practical user needs. By surveying over 200 AI explanation papers and comparing with summarized real-world user demands, we observe two dominating findings: i) humans request diverse XAI questions across the AI pipeline to gain a global view of AI system, whereas existing XAI approaches commonly display a single AI explanation that can not satisfy diverse XAI user needs; ii) humans are widely interested in understanding what AI systems can not achieve, which might lead to the need of interactive AI explanations that enable humans to specify the counterfactual predictions. In light of these findings, we deeply deem that, instead of designating user demands by XAI researchers during AI system development, empowering users to communicate with AI systems for their practical XAI demands is critical to unleashing useful AI explanations (RQ3). To this end, we developed an interactive XAI system via conversations that improved the usefulness of AI explanations in terms of human-perceived performance in AI-assisted writing tasks. Overall, we summarize this doctoral research by discussing the limitations and challenges of human-centered useful AI explanations.
Interpretable Machine Learning
Author | : Christoph Molnar |
Publsiher | : Lulu.com |
Total Pages | : 320 |
Release | : 2020 |
Genre | : Artificial intelligence |
ISBN | : 9780244768522 |
Download Interpretable Machine Learning Book in PDF, Epub and Kindle
This book is about making machine learning models and their decisions interpretable. After exploring the concepts of interpretability, you will learn about simple, interpretable models such as decision trees, decision rules and linear regression. Later chapters focus on general model-agnostic methods for interpreting black box models like feature importance and accumulated local effects and explaining individual predictions with Shapley values and LIME. All interpretation methods are explained in depth and discussed critically. How do they work under the hood? What are their strengths and weaknesses? How can their outputs be interpreted? This book will enable you to select and correctly apply the interpretation method that is most suitable for your machine learning project.
Explainable AI Interpreting Explaining and Visualizing Deep Learning
Author | : Wojciech Samek,Grégoire Montavon,Andrea Vedaldi,Lars Kai Hansen,Klaus-Robert Müller |
Publsiher | : Springer |
Total Pages | : 439 |
Release | : 2019-08-30 |
Genre | : Computers |
ISBN | : 3030289532 |
Download Explainable AI Interpreting Explaining and Visualizing Deep Learning Book in PDF, Epub and Kindle
The development of “intelligent” systems that can take decisions and perform autonomously might lead to faster and more consistent decisions. A limiting factor for a broader adoption of AI technology is the inherent risks that come with giving up human control and oversight to “intelligent” machines. For sensitive tasks involving critical infrastructures and affecting human well-being or health, it is crucial to limit the possibility of improper, non-robust and unsafe decisions and actions. Before deploying an AI system, we see a strong need to validate its behavior, and thus establish guarantees that it will continue to perform as expected when deployed in a real-world environment. In pursuit of that objective, ways for humans to verify the agreement between the AI decision structure and their own ground-truth knowledge have been explored. Explainable AI (XAI) has developed as a subfield of AI, focused on exposing complex AI models to humans in a systematic and interpretable manner. The 22 chapters included in this book provide a timely snapshot of algorithms, theory, and applications of interpretable and explainable AI and AI techniques that have been proposed recently reflecting the current discourse in this field and providing directions of future development. The book is organized in six parts: towards AI transparency; methods for interpreting AI systems; explaining the decisions of AI systems; evaluating interpretability and explanations; applications of explainable AI; and software for explainable AI.
Explainable Human AI Interaction
Author | : Sarath Sarath Sreedharan,Anagha Anagha Kulkarni |
Publsiher | : Springer Nature |
Total Pages | : 164 |
Release | : 2022-05-31 |
Genre | : Computers |
ISBN | : 9783031037672 |
Download Explainable Human AI Interaction Book in PDF, Epub and Kindle
From its inception, artificial intelligence (AI) has had a rather ambivalent relationship with humans—swinging between their augmentation and replacement. Now, as AI technologies enter our everyday lives at an ever-increasing pace, there is a greater need for AI systems to work synergistically with humans. One critical requirement for such synergistic human‒AI interaction is that the AI systems' behavior be explainable to the humans in the loop. To do this effectively, AI agents need to go beyond planning with their own models of the world, and take into account the mental model of the human in the loop. At a minimum, AI agents need approximations of the human's task and goal models, as well as the human's model of the AI agent's task and goal models. The former will guide the agent to anticipate and manage the needs, desires and attention of the humans in the loop, and the latter allow it to act in ways that are interpretable to humans (by conforming to their mental models of it), and be ready to provide customized explanations when needed. The authors draw from several years of research in their lab to discuss how an AI agent can use these mental models to either conform to human expectations or change those expectations through explanatory communication. While the focus of the book is on cooperative scenarios, it also covers how the same mental models can be used for obfuscation and deception. The book also describes several real-world application systems for collaborative decision-making that are based on the framework and techniques developed here. Although primarily driven by the authors' own research in these areas, every chapter will provide ample connections to relevant research from the wider literature. The technical topics covered in the book are self-contained and are accessible to readers with a basic background in AI.
Explainable AI Foundations Methodologies and Applications
Author | : Mayuri Mehta,Vasile Palade,Indranath Chatterjee |
Publsiher | : Springer Nature |
Total Pages | : 273 |
Release | : 2022-10-19 |
Genre | : Technology & Engineering |
ISBN | : 9783031128073 |
Download Explainable AI Foundations Methodologies and Applications Book in PDF, Epub and Kindle
This book presents an overview and several applications of explainable artificial intelligence (XAI). It covers different aspects related to explainable artificial intelligence, such as the need to make the AI models interpretable, how black box machine/deep learning models can be understood using various XAI methods, different evaluation metrics for XAI, human-centered explainable AI, and applications of explainable AI in health care, security surveillance, transportation, among other areas. The book is suitable for students and academics aiming to build up their background on explainable AI and can guide them in making machine/deep learning models more transparent. The book can be used as a reference book for teaching a graduate course on artificial intelligence, applied machine learning, or neural networks. Researchers working in the area of AI can use this book to discover the recent developments in XAI. Besides its use in academia, this book could be used by practitioners in AI industries, healthcare industries, medicine, autonomous vehicles, and security surveillance, who would like to develop AI techniques and applications with explanations.
Hands On Explainable AI XAI with Python
Author | : Denis Rothman |
Publsiher | : Packt Publishing Ltd |
Total Pages | : 455 |
Release | : 2020-07-31 |
Genre | : Computers |
ISBN | : 9781800202764 |
Download Hands On Explainable AI XAI with Python Book in PDF, Epub and Kindle
Resolve the black box models in your AI applications to make them fair, trustworthy, and secure. Familiarize yourself with the basic principles and tools to deploy Explainable AI (XAI) into your apps and reporting interfaces. Key FeaturesLearn explainable AI tools and techniques to process trustworthy AI resultsUnderstand how to detect, handle, and avoid common issues with AI ethics and biasIntegrate fair AI into popular apps and reporting tools to deliver business value using Python and associated toolsBook Description Effectively translating AI insights to business stakeholders requires careful planning, design, and visualization choices. Describing the problem, the model, and the relationships among variables and their findings are often subtle, surprising, and technically complex. Hands-On Explainable AI (XAI) with Python will see you work with specific hands-on machine learning Python projects that are strategically arranged to enhance your grasp on AI results analysis. You will be building models, interpreting results with visualizations, and integrating XAI reporting tools and different applications. You will build XAI solutions in Python, TensorFlow 2, Google Cloud’s XAI platform, Google Colaboratory, and other frameworks to open up the black box of machine learning models. The book will introduce you to several open-source XAI tools for Python that can be used throughout the machine learning project life cycle. You will learn how to explore machine learning model results, review key influencing variables and variable relationships, detect and handle bias and ethics issues, and integrate predictions using Python along with supporting the visualization of machine learning models into user explainable interfaces. By the end of this AI book, you will possess an in-depth understanding of the core concepts of XAI. What you will learnPlan for XAI through the different stages of the machine learning life cycleEstimate the strengths and weaknesses of popular open-source XAI applicationsExamine how to detect and handle bias issues in machine learning dataReview ethics considerations and tools to address common problems in machine learning dataShare XAI design and visualization best practicesIntegrate explainable AI results using Python modelsUse XAI toolkits for Python in machine learning life cycles to solve business problemsWho this book is for This book is not an introduction to Python programming or machine learning concepts. You must have some foundational knowledge and/or experience with machine learning libraries such as scikit-learn to make the most out of this book. Some of the potential readers of this book include: Professionals who already use Python for as data science, machine learning, research, and analysisData analysts and data scientists who want an introduction into explainable AI tools and techniquesAI Project managers who must face the contractual and legal obligations of AI Explainability for the acceptance phase of their applications
Human Computer Interaction INTERACT 2023
Author | : José Abdelnour Nocera,Marta Kristín Lárusdóttir,Helen Petrie,Antonio Piccinno,Marco Winckler |
Publsiher | : Springer Nature |
Total Pages | : 669 |
Release | : 2023-09-25 |
Genre | : Computers |
ISBN | : 9783031422805 |
Download Human Computer Interaction INTERACT 2023 Book in PDF, Epub and Kindle
The four-volume set LNCS 14442 -14445 constitutes the proceedings of the 19th IFIP TC 13 International Conference on Human-Computer Interaction, INTERACT 2023, held in York, UK, in August/September 2023. The 71 full papers and 58 short papers included in this book were carefully reviewed and selected from 406 submissions. They were organized in topical sections as follows: 3D Interaction; Accessibility; Accessibility and Aging; Accessibility for Auditory/Hearing Disabilities; Co-Design; Cybersecurity and Trust; Data Physicalisation and Cross-device; Eye-Free, Gesture Interaction and Sign Language; Haptic interaction and Healthcare applications; Self-Monitoring; Human-Robot Interaction; Information Visualization; Information Visualization and 3D Interaction; Interacting with Children; Interaction with Conversational Agents; Methodologies for HCI; Model-Based UI Design and Testing; Montion Sickness, Stress and Risk perception in 3D Environments and Multisensory interaction; VR experiences; Natural Language Processing and AI Explainability; Online Collaboration and Cooperative work; Recommendation Systems and AI Explainability; Social AI; Social and Ubiquitous Computing; Social Media and Digital Learning; Understanding Users and Privacy Issues; User movement and 3D Environments; User Self-Report; User Studies; User Studies, Eye-Tracking, and Physiological Data; Virtual Reality; Virtual Reality and Training; Courses; Industrial Experiences; Interactive Demonstrations; Keynotes; Panels; Posters; and Workshops.
Explainable Human AI Interaction
Author | : Sarath Sreedharan,Anagha Kulkarni,Subbarao Kambhampati |
Publsiher | : Morgan & Claypool Publishers |
Total Pages | : 184 |
Release | : 2022-01-24 |
Genre | : Computers |
ISBN | : 9781636392905 |
Download Explainable Human AI Interaction Book in PDF, Epub and Kindle
From its inception, artificial intelligence (AI) has had a rather ambivalent relationship with humans—swinging between their augmentation and replacement. Now, as AI technologies enter our everyday lives at an ever-increasing pace, there is a greater need for AI systems to work synergistically with humans. One critical requirement for such synergistic human‒AI interaction is that the AI systems' behavior be explainable to the humans in the loop. To do this effectively, AI agents need to go beyond planning with their own models of the world, and take into account the mental model of the human in the loop. At a minimum, AI agents need approximations of the human's task and goal models, as well as the human's model of the AI agent's task and goal models. The former will guide the agent to anticipate and manage the needs, desires and attention of the humans in the loop, and the latter allow it to act in ways that are interpretable to humans (by conforming to their mental models of it), and be ready to provide customized explanations when needed. The authors draw from several years of research in their lab to discuss how an AI agent can use these mental models to either conform to human expectations or change those expectations through explanatory communication. While the focus of the book is on cooperative scenarios, it also covers how the same mental models can be used for obfuscation and deception. The book also describes several real-world application systems for collaborative decision-making that are based on the framework and techniques developed here. Although primarily driven by the authors' own research in these areas, every chapter will provide ample connections to relevant research from the wider literature. The technical topics covered in the book are self-contained and are accessible to readers with a basic background in AI.