Modeling Techniques In Predictive Analystics With Python And R
Download Modeling Techniques In Predictive Analystics With Python And R full books in PDF, epub, and Kindle. Read online free Modeling Techniques In Predictive Analystics With Python And R ebook anywhere anytime directly on your device. Fast Download speed and no annoying ads. We cannot guarantee that every ebooks is available!
Modeling Techniques in Predictive Analytics with Python and R
Author | : Thomas W. Miller |
Publsiher | : FT Press |
Total Pages | : 448 |
Release | : 2014-09-29 |
Genre | : Computers |
ISBN | : 9780133892147 |
Download Modeling Techniques in Predictive Analytics with Python and R Book in PDF, Epub and Kindle
Master predictive analytics, from start to finish Start with strategy and management Master methods and build models Transform your models into highly-effective code—in both Python and R This one-of-a-kind book will help you use predictive analytics, Python, and R to solve real business problems and drive real competitive advantage. You’ll master predictive analytics through realistic case studies, intuitive data visualizations, and up-to-date code for both Python and R—not complex math. Step by step, you’ll walk through defining problems, identifying data, crafting and optimizing models, writing effective Python and R code, interpreting results, and more. Each chapter focuses on one of today’s key applications for predictive analytics, delivering skills and knowledge to put models to work—and maximize their value. Thomas W. Miller, leader of Northwestern University’s pioneering program in predictive analytics, addresses everything you need to succeed: strategy and management, methods and models, and technology and code. If you’re new to predictive analytics, you’ll gain a strong foundation for achieving accurate, actionable results. If you’re already working in the field, you’ll master powerful new skills. If you’re familiar with either Python or R, you’ll discover how these languages complement each other, enabling you to do even more. All data sets, extensive Python and R code, and additional examples available for download at http://www.ftpress.com/miller/ Python and R offer immense power in predictive analytics, data science, and big data. This book will help you leverage that power to solve real business problems, and drive real competitive advantage. Thomas W. Miller’s unique balanced approach combines business context and quantitative tools, illuminating each technique with carefully explained code for the latest versions of Python and R. If you’re new to predictive analytics, Miller gives you a strong foundation for achieving accurate, actionable results. If you’re already a modeler, programmer, or manager, you’ll learn crucial skills you don’t already have. Using Python and R, Miller addresses multiple business challenges, including segmentation, brand positioning, product choice modeling, pricing research, finance, sports, text analytics, sentiment analysis, and social network analysis. He illuminates the use of cross-sectional data, time series, spatial, and spatio-temporal data. You’ll learn why each problem matters, what data are relevant, and how to explore the data you’ve identified. Miller guides you through conceptually modeling each data set with words and figures; and then modeling it again with realistic code that delivers actionable insights. You’ll walk through model construction, explanatory variable subset selection, and validation, mastering best practices for improving out-of-sample predictive performance. Miller employs data visualization and statistical graphics to help you explore data, present models, and evaluate performance. Appendices include five complete case studies, and a detailed primer on modern data science methods. Use Python and R to gain powerful, actionable, profitable insights about: Advertising and promotion Consumer preference and choice Market baskets and related purchases Economic forecasting Operations management Unstructured text and language Customer sentiment Brand and price Sports team performance And much more
Marketing Data Science
Author | : Thomas W. Miller |
Publsiher | : FT Press |
Total Pages | : 810 |
Release | : 2015-05-02 |
Genre | : Business & Economics |
ISBN | : 9780133887341 |
Download Marketing Data Science Book in PDF, Epub and Kindle
Now, a leader of Northwestern University's prestigious analytics program presents a fully-integrated treatment of both the business and academic elements of marketing applications in predictive analytics. Writing for both managers and students, Thomas W. Miller explains essential concepts, principles, and theory in the context of real-world applications. Building on Miller's pioneering program, Marketing Data Science thoroughly addresses segmentation, target marketing, brand and product positioning, new product development, choice modeling, recommender systems, pricing research, retail site selection, demand estimation, sales forecasting, customer retention, and lifetime value analysis. Starting where Miller's widely-praised Modeling Techniques in Predictive Analytics left off, he integrates crucial information and insights that were previously segregated in texts on web analytics, network science, information technology, and programming. Coverage includes: The role of analytics in delivering effective messages on the web Understanding the web by understanding its hidden structures Being recognized on the web – and watching your own competitors Visualizing networks and understanding communities within them Measuring sentiment and making recommendations Leveraging key data science methods: databases/data preparation, classical/Bayesian statistics, regression/classification, machine learning, and text analytics Six complete case studies address exceptionally relevant issues such as: separating legitimate email from spam; identifying legally-relevant information for lawsuit discovery; gleaning insights from anonymous web surfing data, and more. This text's extensive set of web and network problems draw on rich public-domain data sources; many are accompanied by solutions in Python and/or R. Marketing Data Science will be an invaluable resource for all students, faculty, and professional marketers who want to use business analytics to improve marketing performance.
Modeling Techniques in Predictive Analytics
Author | : Thomas W. Miller |
Publsiher | : FT Press |
Total Pages | : 376 |
Release | : 2014-09-29 |
Genre | : Business & Economics |
ISBN | : 9780133886191 |
Download Modeling Techniques in Predictive Analytics Book in PDF, Epub and Kindle
To succeed with predictive analytics, you must understand it on three levels: Strategy and management Methods and models Technology and code This up-to-the-minute reference thoroughly covers all three categories. Now fully updated, this uniquely accessible book will help you use predictive analytics to solve real business problems and drive real competitive advantage. If you’re new to the discipline, it will give you the strong foundation you need to get accurate, actionable results. If you’re already a modeler, programmer, or manager, it will teach you crucial skills you don’t yet have. Unlike competitive books, this guide illuminates the discipline through realistic vignettes and intuitive data visualizations–not complex math. Thomas W. Miller, leader of Northwestern University’s pioneering program in predictive analytics, guides you through defining problems, identifying data, crafting and optimizing models, writing effective R code, interpreting results, and more. Every chapter focuses on one of today’s key applications for predictive analytics, delivering skills and knowledge to put models to work–and maximize their value. Reflecting extensive student and instructor feedback, this edition adds five classroom-tested case studies, updates all code for new versions of R, explains code behavior more clearly and completely, and covers modern data science methods even more effectively. All data sets, extensive R code, and additional examples available for download at http://www.ftpress.com/miller If you want to make the most of predictive analytics, data science, and big data, this is the book for you. Thomas W. Miller’s unique balanced approach combines business context and quantitative tools, appealing to managers, analysts, programmers, and students alike. Miller addresses multiple business cases and challenges, including segmentation, brand positioning, product choice modeling, pricing research, finance, sports, text analytics, sentiment analysis, and social network analysis. He illuminates the use of cross-sectional data, time series, spatial, and spatio-temporal data. You’ll learn why each problem matters, what data are relevant, and how to explore the data you’ve identified. Miller guides you through conceptually modeling each data set with words and figures; and then modeling it again with realistic R programs that deliver actionable insights. You’ll walk through model construction, explanatory variable subset selection, and validation, mastering best practices for improving out-of-sample predictive performance. Throughout, Miller employs data visualization and statistical graphics to help you explore data, present models, and evaluate performance. This edition adds five new case studies, updates all code for the newest versions of R, adds more commenting to clarify how the code works, and offers a more detailed and up-to-date primer on data science methods. Gain powerful, actionable, profitable insights about: Advertising and promotion Consumer preference and choice Market baskets and related purchases Economic forecasting Operations management Unstructured text and language Customer sentiment Brand and price Sports team performance And much more
Marketing Data Science
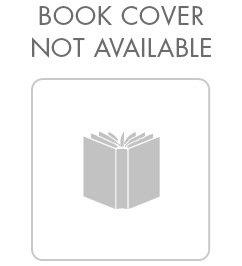
Author | : Thomas W. Miller |
Publsiher | : Unknown |
Total Pages | : 135 |
Release | : 2015 |
Genre | : Data mining |
ISBN | : 0133887626 |
Download Marketing Data Science Book in PDF, Epub and Kindle
Modeling Techniques in Predictive Analystics with Python and R
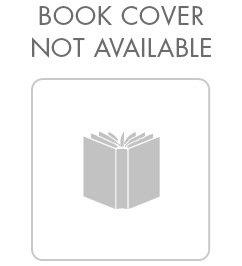
Author | : Thomas W. Miller |
Publsiher | : Unknown |
Total Pages | : 418 |
Release | : 2014 |
Genre | : Business planning |
ISBN | : 0133892123 |
Download Modeling Techniques in Predictive Analystics with Python and R Book in PDF, Epub and Kindle
Extending Power BI with Python and R
Author | : Luca Zavarella,Francesca Lazzeri |
Publsiher | : Packt Publishing Ltd |
Total Pages | : 559 |
Release | : 2021-11-26 |
Genre | : Computers |
ISBN | : 9781801076678 |
Download Extending Power BI with Python and R Book in PDF, Epub and Kindle
Perform more advanced analysis and manipulation of your data beyond what Power BI can do to unlock valuable insights using Python and R Key FeaturesGet the most out of Python and R with Power BI by implementing non-trivial codeLeverage the toolset of Python and R chunks to inject scripts into your Power BI dashboardsImplement new techniques for ingesting, enriching, and visualizing data with Python and R in Power BIBook Description Python and R allow you to extend Power BI capabilities to simplify ingestion and transformation activities, enhance dashboards, and highlight insights. With this book, you'll be able to make your artifacts far more interesting and rich in insights using analytical languages. You'll start by learning how to configure your Power BI environment to use your Python and R scripts. The book then explores data ingestion and data transformation extensions, and advances to focus on data augmentation and data visualization. You'll understand how to import data from external sources and transform them using complex algorithms. The book helps you implement personal data de-identification methods such as pseudonymization, anonymization, and masking in Power BI. You'll be able to call external APIs to enrich your data much more quickly using Python programming and R programming. Later, you'll learn advanced Python and R techniques to perform in-depth analysis and extract valuable information using statistics and machine learning. You'll also understand the main statistical features of datasets by plotting multiple visual graphs in the process of creating a machine learning model. By the end of this book, you'll be able to enrich your Power BI data models and visualizations using complex algorithms in Python and R. What you will learnDiscover best practices for using Python and R in Power BI productsUse Python and R to perform complex data manipulations in Power BIApply data anonymization and data pseudonymization in Power BILog data and load large datasets in Power BI using Python and REnrich your Power BI dashboards using external APIs and machine learning modelsExtract insights from your data using linear optimization and other algorithmsHandle outliers and missing values for multivariate and time-series dataCreate any visualization, as complex as you want, using R scriptsWho this book is for This book is for business analysts, business intelligence professionals, and data scientists who already use Microsoft Power BI and want to add more value to their analysis using Python and R. Working knowledge of Power BI is required to make the most of this book. Basic knowledge of Python and R will also be helpful.
Hands On Predictive Analytics with Python
Author | : Alvaro Fuentes |
Publsiher | : Packt Publishing Ltd |
Total Pages | : 320 |
Release | : 2018-12-28 |
Genre | : Computers |
ISBN | : 9781789134544 |
Download Hands On Predictive Analytics with Python Book in PDF, Epub and Kindle
Step-by-step guide to build high performing predictive applications Key FeaturesUse the Python data analytics ecosystem to implement end-to-end predictive analytics projectsExplore advanced predictive modeling algorithms with an emphasis on theory with intuitive explanationsLearn to deploy a predictive model's results as an interactive applicationBook Description Predictive analytics is an applied field that employs a variety of quantitative methods using data to make predictions. It involves much more than just throwing data onto a computer to build a model. This book provides practical coverage to help you understand the most important concepts of predictive analytics. Using practical, step-by-step examples, we build predictive analytics solutions while using cutting-edge Python tools and packages. The book's step-by-step approach starts by defining the problem and moves on to identifying relevant data. We will also be performing data preparation, exploring and visualizing relationships, building models, tuning, evaluating, and deploying model. Each stage has relevant practical examples and efficient Python code. You will work with models such as KNN, Random Forests, and neural networks using the most important libraries in Python's data science stack: NumPy, Pandas, Matplotlib, Seaborn, Keras, Dash, and so on. In addition to hands-on code examples, you will find intuitive explanations of the inner workings of the main techniques and algorithms used in predictive analytics. By the end of this book, you will be all set to build high-performance predictive analytics solutions using Python programming. What you will learnGet to grips with the main concepts and principles of predictive analyticsLearn about the stages involved in producing complete predictive analytics solutionsUnderstand how to define a problem, propose a solution, and prepare a datasetUse visualizations to explore relationships and gain insights into the datasetLearn to build regression and classification models using scikit-learnUse Keras to build powerful neural network models that produce accurate predictionsLearn to serve a model's predictions as a web applicationWho this book is for This book is for data analysts, data scientists, data engineers, and Python developers who want to learn about predictive modeling and would like to implement predictive analytics solutions using Python's data stack. People from other backgrounds who would like to enter this exciting field will greatly benefit from reading this book. All you need is to be proficient in Python programming and have a basic understanding of statistics and college-level algebra.
Applied Predictive Modeling
Author | : Max Kuhn,Kjell Johnson |
Publsiher | : Springer Science & Business Media |
Total Pages | : 600 |
Release | : 2013-05-17 |
Genre | : Medical |
ISBN | : 9781461468493 |
Download Applied Predictive Modeling Book in PDF, Epub and Kindle
Applied Predictive Modeling covers the overall predictive modeling process, beginning with the crucial steps of data preprocessing, data splitting and foundations of model tuning. The text then provides intuitive explanations of numerous common and modern regression and classification techniques, always with an emphasis on illustrating and solving real data problems. The text illustrates all parts of the modeling process through many hands-on, real-life examples, and every chapter contains extensive R code for each step of the process. This multi-purpose text can be used as an introduction to predictive models and the overall modeling process, a practitioner’s reference handbook, or as a text for advanced undergraduate or graduate level predictive modeling courses. To that end, each chapter contains problem sets to help solidify the covered concepts and uses data available in the book’s R package. This text is intended for a broad audience as both an introduction to predictive models as well as a guide to applying them. Non-mathematical readers will appreciate the intuitive explanations of the techniques while an emphasis on problem-solving with real data across a wide variety of applications will aid practitioners who wish to extend their expertise. Readers should have knowledge of basic statistical ideas, such as correlation and linear regression analysis. While the text is biased against complex equations, a mathematical background is needed for advanced topics.